Page overview
Reducing packaging use
The Packaging Decision Engine is an AI model helping determine the most efficient packaging options to ship millions of items available to Amazon customers. Data scientists have trained the model to understand a variety of product attributes, including an item’s shape and durability, and to analyze customer feedback on how different packaging options have performed. The model is constantly learning and has helped reduce the company’s use of packaging material since it launched in 2019. With this and other packaging innovations, Amazon has eliminated over two million tons of packaging material worldwide since 2015.
AI-powered technology is being used across a growing number of fulfillment centers to detect damaged goods, with the goal of decreasing the number of damaged items that get sent to and returned by customers. The AI is three times more effective at identifying damaged goods than human beings and has been trained by analyzing millions of photos of undamaged and damaged items. If a product can’t be shipped directly to a customer due to imperfections, the item is flagged to an Amazon associate, who assesses the product and reroutes it to be resold at a reduced price, donated, or otherwise reused.
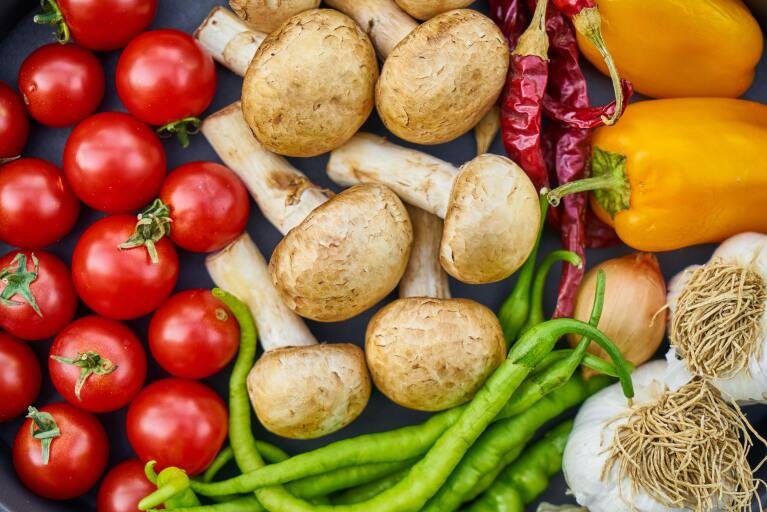
A growing number of Amazon Fresh grocery teams are using machine learning-based solutions to automate store shelf monitoring for fruits and vegetables. This AI-powered solution analyzes crate images to detect visual imperfections on the produce like cracks, cuts, and pressure damage. To ensure the defective produce is recycled whenever possible, Amazon Fresh resells the usable produce to local contractors who further resell the produce at reduced prices for use cases like feeding to livestock, ensuring less food goes to waste.
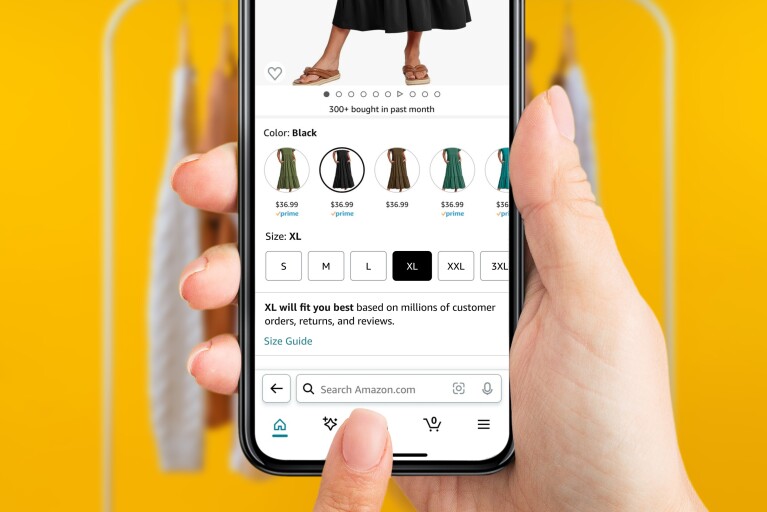
Reducing returns leads to more sustainable shopping. Amazon introduced several AI-powered innovations to help customers shop for fashion with more confidence in Amazon’s store, while also helping reduce fit-related returns. They include personalized size recommendations using AI and ML to help customers find what size fits them best, personalized feedback from customers who wear the same size, and improved size charts. Amazon also developed a Fit Insights Tool to help brands and selling partners better understand customer fit issues and incorporate feedback into future designs and manufacturing, helping brands more accurately list their items for customers and reduce fit-related returns.
Estimating the carbon footprint for millions of Amazon products can be challenging—it can take a person hundreds of hours to research and calculate the carbon footprint for even a single product. To solve that challenge, Amazon developed Flamingo, an AI-based algorithm that leverages natural language processing to match text descriptions for Environmental Impact Factors (EIF)—a commonly accepted measurement for calculating the carbon impact of an item—with specific products.
The algorithm is already helping Amazon’s team calculate the environmental impacts of everything from cotton t-shirts sold by Amazon Private Brands to carrots sold by Amazon Fresh. In one experiment, the algorithm reduced the time scientists spent mapping 15,000 Amazon products from a month down to several hours. Flamingo is also available for other companies to use in order to help accelerate their sustainability goals.
Amazon democratizes AI so other companies can use it to help meet their own sustainability goals. As one example, AWS worked with a Brazilian nonprofit to develop a large-scale AI model that monitors deforestation. This has enabled automatic monitoring of 20 million hectares of forest areas. With better monitoring, it’s estimated that 3.4 million hectares of forested areas will be restored within the state of Para.
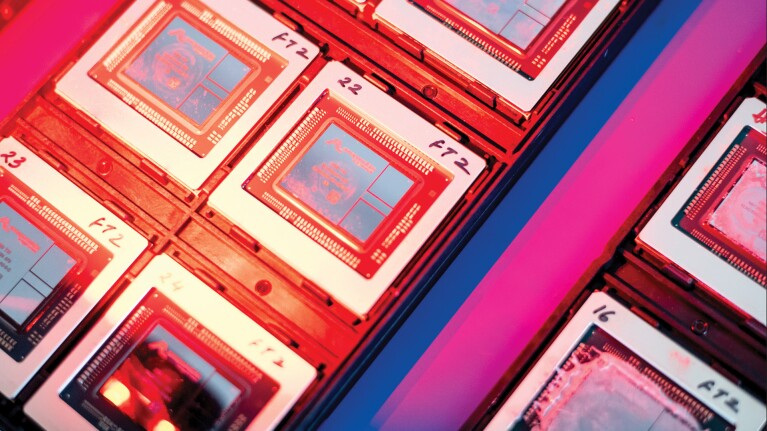
Amazon is also improving the sustainability of AI by making our cloud infrastructure more energy efficient, including by investing in AWS chips. AWS Trainium is a high-performance machine learning chip designed to reduce the time and cost of training generative AI models—cutting training time for some models from months to hours. This means building new models requires less money and power, with potential cost savings of up to 50% and energy-consumption reductions of up to 29%, versus comparable instances.
Our second-generation Trainium2 chips are designed to deliver up to four times faster training than first-generation Trainium chips while improving energy efficiency up to two times. AWS Inferentia is our most power-efficient AI inference chip. Our Inferentia2 AI accelerator delivers up to 50% higher performance per watt and can reduce costs by up to 40% against comparable instances.
Trending news and stories
- 3 things you didn’t know about Ring
- Meet the cast and characters of ‘Reacher’ Season 3, premiering February 20 on Prime Video
- How to watch the third and final season of ‘Bosch: Legacy’ on Prime Video
- Amazon Prime members enjoyed fastest-ever delivery speeds in 2024, and US members saved on average over $500 on delivery fees