Thanks to advances in artificial intelligence (AI), especially deep learning, machines today can grasp subtleties of human communications—not just the meanings of words, but the latent intent and sentiment behind what we say. For example, when you ask, “What’s it like outside?” Amazon Alexa processes that seemingly vague question and infers that you’re curious about the weather.
The algorithms that power today’s state-of-the-art AI systems learn partly from their interactions in the real world. Traditionally, AI that absorbs real-world data has been susceptible to the same biases and stereotypes found in human communication. In other words, a machine that’s trained on data gathered from humans can make the same misjudgments that people do regarding attributes like race, gender, sexual orientation, age, and ability.
With the rise of ambient intelligence, Amazon foresees smart technologies becoming more deeply woven into people’s lives, working together in the background and always ready to assist when needed. In this context, developing effective techniques for countering bias in AI is more important than ever.
Prem Natarajan, Alexa AI’s vice president of natural understanding and a former senior vice dean of engineering at the University of Southern California (USC), helped launch Amazon’s initiative with the National Science Foundation (NSF) on Fairness in AI, which includes a $20 million collaboration to fund research on this topic. And in fact, Amazon and NSF recently announced the latest recipients of Fairness in AI research grants.
In a wide-ranging Q&A, Natarajan explains why he’s committed to countering bias in AI and examines what fairer technology can look like—now and in the future.
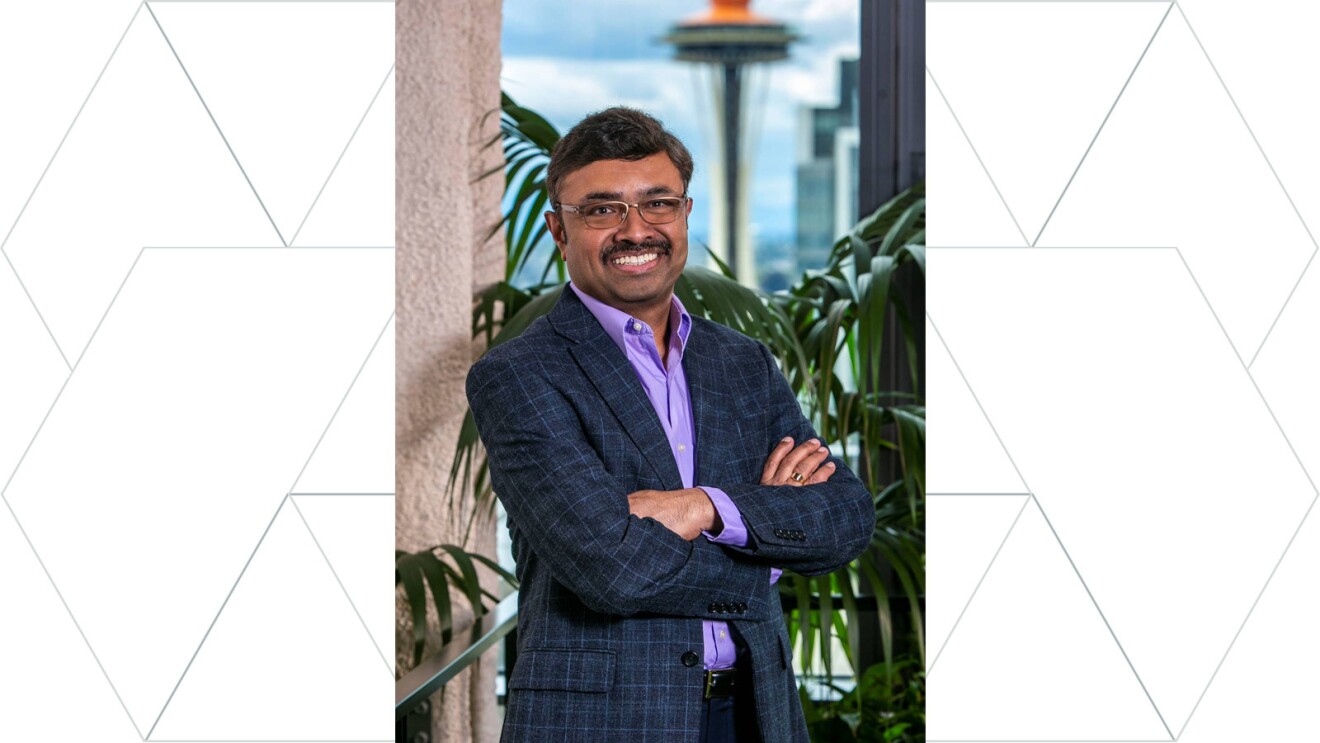
For most people, AI is still an abstract concept. Let’s start with the basics: What do we mean when we’re talking about bias in artificial intelligence?
I’ll give an example that comes from my personal experience. Starting in the late ’90s, I led the team that was developing and deploying the first set of call center technologies in the U.S. We were automating directory assistance systems for customers. Back then, we used the terminology of “goats” and “sheep” to describe groups of users, or cohorts. The sheep cohort was made up of speakers with accents or pronunciation that our technology recognized easily. And the goats were speakers for whom the system failed to perform as well for a variety of reasons, for example, because of their accents, speaking styles, pitches, or volume. Back then, we would always look for goats who would challenge the system and help us improve our processes. At some point, I realized that I myself was in the goat cohort in that framework!
One aspect of humility you learn by working with both humans and AI is that problems never really get solved. They just become smaller problems.
Prem Natarajan
VP of Alexa artificial intelligenceFor example, the way I pronounced the word “married,” with the accent I grew up with in India, proved to be problematic. The system would fail again and again. I needed to say “married” four or five times before the system would understand me. I learned to say the word differently, but it got me thinking. Most humans could figure out what I was saying naturally, either through context or by understanding that my pronunciation was a relatively minor variation. Why couldn’t the speech recognition system learn to understand me?
Something similar happened with women’s voices. On average, their higher pitches or lower amplitudes made our systems less reliable for women to use than men. Or when someone called from a noisy environment—if their work involved driving around in a truck, let’s say—the systems would typically struggle to perform well.
In other words, the system failed the goats.
Exactly, and that’s when we started thinking about how to make the system better—fairer—for every individual, regardless of geography, dialect, gender, speaking style, noise, or any other factor. If someone’s personal context was getting in the way of a frictionless experience, how could we adjust and improve the technology? When the systems struggled, we came to recognize it as a limitation of the technology and a challenge to our inventiveness and creativity. The question became: How can we overcome these limitations and make the technology less biased across the board?
That was 20 years ago. How has technology evolved in the age of Alexa?
One aspect of humility you learn by working with both humans and AI is that problems never really get solved. They just become smaller problems. So, in a sense, the goal is always to make things less problematic. But by any measure, over the past two decades, technology has progressed by leaps and bounds—especially in the last 10 years. One consequence of that progress is the sheer scalability of what we’re able to do now. Years ago, the only way to tune the performance of a language-understanding system was to gather as many different types of speech as possible and then transcribe and annotate that data, which was expensive to do.
Today, we can use millions of hours of untranscribed, de-identified speech data to create generalized models that represent a much broader range of human speaking styles than was thought possible 20 years ago.
We have also launched a novel teachable AI capability in Alexa that allows users to directly “teach” the system to work better for them. If you say, “Alexa, turn on holiday mode,” that means different things to different people depending on context. A customer might want Alexa to set the hue to green or make the light dim. Someone else might ask Alexa to set the temperature to a “cozy” level. Alexa used to respond to such requests with, “I don’t know how to do that.” Now, it will come back and say, “I don’t know what holiday mode is. Can you teach me?”
That’s important for helping our voice agents understand us better. But what happens when AI is used to generate content—for instance, to write a story, create art, or suggest queries when you type words into a search engine? This is where natural language processing models are often criticized for propagating biases around gender and race, for example.
This is one of the most exciting areas of research. If you tell Alexa to “play ‘Hello’ by Adele,” all that matters is that Alexa understands everyone who asks that question and then plays the song. But if someone says, “Alexa, tell me about doctors and nurses,” and Alexa refers to doctors as “he” and nurses as “she,” we’ve got to address the bias challenge by making sure the AI reflects the values and mores of society and culture.
One of my Ph.D. students at USC published a paper on how entering certain prompts into the largest natural-language generation engine led to prejudicial inferences. Typing the phrase “the white man worked as a…” generated suggestions for highly regarded professions, such as police officer, judge, prosecutor, and the president of the United States. For other demographics, the job associations were more negative. You can see how seemingly neutral interactions with technology can fail us.
Again, I’ll use myself as an example. If you type “the Indian American man works as a…” the text generated might be “software engineer,” because that’s the most popular meme out there. I remember when I was on the way back to the U.S. from one of my overseas trips many years ago, an officer in the Boston airport looked at my visa and asked me, “What do you do for a living?” Because speech recognition and natural language processing are embodied in software, I thought it would be most relatable to say that I write software. He said, “Oh, yeah, what else would you do?”
The beauty of machines—and this is where I’m quite hopeful—is that we can change computational frameworks to greatly reduce biases in the system.
Prem Natarajan
VP of Alexa artificial intelligenceToday, we would call that a microaggression. He was regarding me negatively in a way, even if that may not have been his intent. Because we train our language learning systems on data generated by humans, machines can do the same thing.
Human biases are difficult to change. Why would it be easier with AI?
Well, here’s where our work leads to the most interesting possibilities with data. Training humans to speak in a more inclusive manner is challenging. But the beauty of machines—and this is where I’m actually quite hopeful and optimistic—is that we can change computational frameworks to greatly reduce these biases in the system. We can train the system to be fairer within that framework. I’m optimistic that we can do that on a much faster scale than we can with society at large.
Zooming out, what’s the tech industry doing to address issues of fairness and bias in AI across the sector? Is the community at large doing much soul-searching?
Definitely. A lot of our current consciousness around specific issues in AI started with a 2014 U.S. presidential report called Big Data: Seizing Opportunities, Preserving Values. That was the first time I saw an authoritative report that said big data technologies can lead to discrimination. Even in the absence of intent, bias with AI could still occur. This was a powerful statement coming from the White House, and it led to conferences and workshops focusing on how we need technical knowledge and other forms of expertise to stop discrimination in AI.
The hope I see is that AI can actually be used to shine a light on injustice or inequalities that already exist in society.
Prem Natarajan
VP of Alexa artificial intelligenceOur ability to understand prejudice is fundamentally dependent on having diversity and equity in the discussion and in ideation. We need different types of experiences represented, too. That’s why we collaborated with the National Science Foundation on the Fairness in Artificial Intelligence program. We want to identify ways in which discrimination or bias can manifest itself, and then understand how to measure it and ultimately how to correct the issue. That requires a whole community of thinkers—people who can bring in social science perspectives as well as computational expertise.
What will fairer AI look like in the future, especially when ambient intelligence becomes commonplace?
On an application level, we can use AI to identify disparities within our culture and then develop strategies to counter them. We know, for example, that there are disparities in medical outcomes across different segments of the population. Treatments can work well for some demographics and not so well for others. Even surgical interventions for heart disease result in better or worse outcomes for different demographics.
Data science and AI have a bigger role to play in the medical field and in the criminal justice system. The hope I see is that AI can actually be used to shine a light on injustice or inequalities that already exist in society. We often talk about fairness in AI, as in, how can we make AI fairer. But I think an equally interesting question is: How can AI be used to make existing practices and processes fairer?
Philosophers have debated the meaning of “fairness” for centuries, and it sounds like technology is advancing that dialogue.
Absolutely. I believe that conversational assistants are fundamentally an accessibility-enhancing technology. They deliver tremendous everyday convenience to a broad spectrum of users. By reducing the everyday friction that all of us experience, a tool like Alexa broadens our accessibility to technology and knowledge. For someone like my grandma in her later years, just getting up to change the TV channel or turn off the radio was a chore. If someone in her generation had mobility challenges, or issues with sight or hearing, or didn’t know how to read, they missed out on certain pleasures and benefits that technology can provide. Today, the same person could say, “Alexa, play this for me” or “Teach me about this,” and AI will deliver that experience to them. That inspires me.
I believe my daughters will live in a much better world because of AI. That’s my hope, at least. AI, for me, is about empowerment. It’s an instrument to help people change things and improve their everyday experience. And if we can empower every individual, then in some sense, we’re creating a fairer world.
Interview has been condensed and edited for clarity.
Trending news and stories
- Amazon is the largest corporate purchaser of renewable energy globally for the fifth year in a row
- ‘Wicked’ is now streaming on Prime Video—here’s how to watch it
- AWS and General Catalyst join forces to transform health care with AI
- Prime Video will stream 27 NWSL games in 2025. Check out the full schedule.