On September 20, at an event at Amazon’s Spheres, Amazon senior vice president for devices and services Dave Limp unveiled dozens of new device products and features. Later that day, Alexa head scientist Rohit Prasad went behind the scenes, explaining the scientific advances underlying Alexa’s newest features and capabilities.
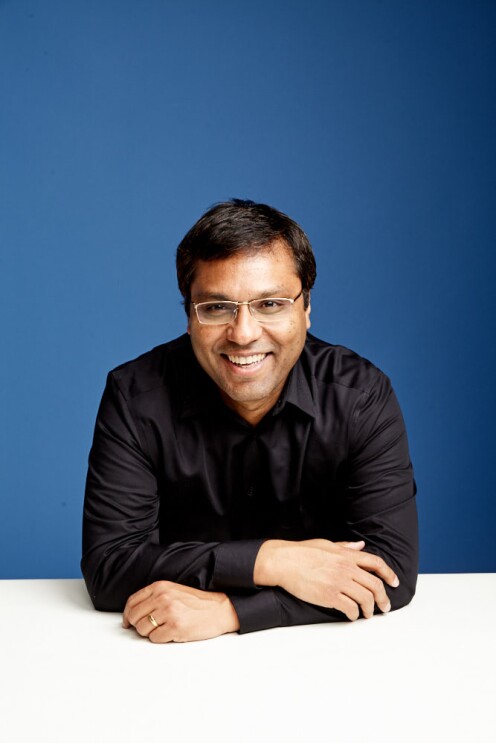
Alexa research and development falls into five major categories, Prasad explained. The first is competence, learning new skills and improving performance on existing ones. The next is context awareness, using information about the state of the world and about customers’ past interactions with Alexa to decide how best to handle a particular request. The third is expanding Alexa’s knowledge of facts and events, and the fourth is enabling more natural interaction with the Alexa voice service. "Being true to our promise to our customer that Alexa gets better every day,” Prasad said, the fifth category is self-learning, or automating the process whereby Alexa learns from experience.
"Most Alexa AI research is driven by machine-learning techniques that leverage large-scale AWS computing power and rich, heterogeneous data sets," explained Prasad. And here's how Alexa researchers are applying those techniques to these five research areas.
Competence
“Alexa features more than 50,000 skills built by third party developers,” Prasad said. “We are helping democratize AI through our Alexa Skills Kit.” At the same time, Prasad said, over the past 12 months, the Alexa team has reduced Alexa’s error rate.
“Because we have had this massive growth in skills,” Prasad said, “just maintaining the accuracy would be great. But the team has gone further and even reduced the error rates in every location and every language Alexa has launched in.”
One of the techniques that enabled that improvement, Prasad explained, is active learning, in which automated systems sort through training data to extract those examples that are likely to yield the most significant improvements in accuracy. Alexa researchers have found that active learning can reduce the amount of data necessary to train a machine learning system by up to 97 percent, Prasad said, enabling much more rapid improvement of Alexa’s natural-language-understanding systems.
Alexa researchers have also made what Prasad described as a “breakthrough” in the rapid development of new deep-learning networks, machine learning systems that consist of thousands or even millions of densely connected (virtual) processing units. This breakthrough combines deep learning for natural-language understanding with transfer learning, in which a network trained to perform a task for which a large set of training data is available is then retrained on a related task, with little available data.
“What this will do is give 15 percent relative improvement in accuracy for custom skills with no additional work from the third-party developer,” Prasad said. “We are rolling this out in the coming months to all skills.”
Context awareness
Alexa already exhibits context awareness by customizing its decisions based on which device a customer is interacting with, Prasad explained. The command “play Hunger Games,” for instance, is more likely to launch a movie on a device with a screen, like the Echo Show, than on a voice-only device, which would instead play the audiobook.
But two of the new features announced in September — the sound detection technology that lets Alexa Guard recognize smoke alarms, carbon monoxide alarms, and glass breaking and whisper detection for Whisper Mode — expand Alexa’s awareness of the customer’s aural context beyond the recognition and understanding of words.
"Both systems use a machine learning network known as a long short-term memory," Prasad explained. Incoming audio signals are broken into ultrashort snippets, and the long short-term memory network processes them in order. Its judgment about any given snippet — is this a whisper? is this an alarm? — factors in its judgments about preceding snippets, so it can learn systematic relationships between segments of an audio signal that are separated in time.
These networks automatically learn the features of audio signals useful for detecting sound events or whispered speech. For instance, they automatically learn the frequency characteristics of whispered speech, rather than relying on features manually engineered for whisper detection.
Knowledge
"In the past 12 months, Amazon’s knowledge team has added billions of data points to Alexa’s knowledge graph, a representation of named entities and their attributes and relationships," explained Prasad.
He also pointed out that, without a single knowledge source that’s authoritative on every subject, Alexa researchers are combining heterogeneous knowledge sources to provide the best answers to customer queries.
Natural interaction
“One of the technologies making voice interaction with Alexa more natural,” Prasad said, “is context carryover, or tracking references through several rounds of conversation.” For instance, a customer might ask, “Alexa, is it going to rain today?”, then follow up by saying, “How about tomorrow?” Alexa can handle this type of ambiguous reference today.
“How we do this is, again, applying long short-term memory networks across different turns to merge hypotheses from previous turns to make the best answer,” Prasad explained.
Alexa is also moving toward what Prasad described as “natural skill interaction”. In the past, customers engaging with Alexa would have to specify the names of the skills they wished to invoke. Now, a machine learning system will automatically select the one skill that best addresses a specific customer request. That system has two components: the first produces a shortlist of candidate skills based on the customer’s request; the second uses more detailed information to choose among the skills on the shortlist.
"Natural skill interaction is available for several thousands of skills in the U.S.," Prasad said, "with worldwide rollouts to follow."
Self-learning
“Going back to our promise to customers," said Prasad, "we want Alexa to learn at a much faster pace."
To increase the rate that Alexa learns from interactions, the Alexa team is developing self-learning techniques, instead of relying on “supervised” training that requires data laboriously annotated by hand.
One such technique, which Alexa will begin using in the coming months, is automatic equivalence class learning, which uses the fact that seasoned Alexa customers will often rephrase requests that initially fail. If an Alexa customer in the Seattle area, for instance, requests the satellite radio station Sirius XM Chill, and that request fails, she might rephrase it as Sirius channel 53. An automated system can recognize that these requests share a word (“Sirius”), that the second request was successful, and that both names should be treated as referring to the same entity.
The future
“We will continue to make Alexa more useful and delightful by shifting the cognitive burden for more complex tasks from our customers to Alexa,” said Prasad. “I’m optimistic that our investments in all layers of our AI stack will continue to make Alexa smarter at a breakneck pace.”
Trending news and stories