A face-off can be one of the most pivotal moments in a hockey game. The winning player can swing the momentum to their team and directly impact the game’s outcome.
Imagine, it’s late in the third period of a close game, and two hockey players are face to face on the ice, waiting for the puck to drop. Everyone is guessing who will get to the puck first. But until now, no one had a comprehensive way to assess who was more likely to win.
To close this gap, the National Hockey League (NHL) and Amazon Web Services (AWS) developed Face-off Probability, which uses AI to deliver real-time face-off predictions to analysts, fans, and teams. This is a significant technology development because it’s the first probability stat from AWS that will be seen live before the play, instead of as it happens.
During a stoppage in play, Face-off Probability generates predictions for who will win the upcoming face-off based on the players on the ice, location of the face-off, and current game situation. The predictions occur in less than a second and are generated throughout the stoppage until the game clock starts running again.
The teams working to tackle the challenge of producing real-time face-off predictions had to build this playbook from scratch. But AWS was uniquely positioned to generate a solution given its record of building data-driven solutions for sports and its deep technology partnership with the NHL, which already produced shot and save analytics in 2021.
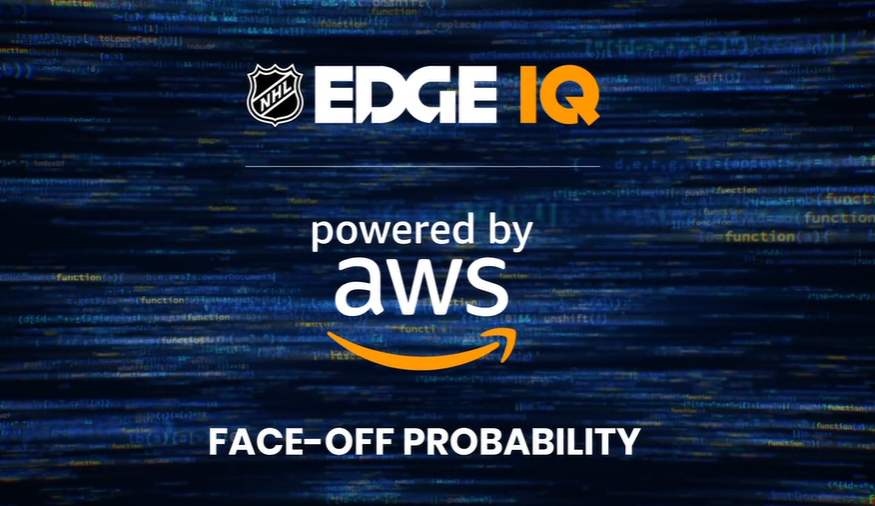
Applying AWS machine learning (ML) to NHL data
Building an entirely new system from scratch that can proactively evaluate face-off matchups and predict winners posed significant technical challenges.
The NHL and AWS used Player, Puck, and Tracking (PPT) data, and hundreds of thousands of face-offs from over 10 years of historical NHL Hockey Information & Tracking System (HITS) stats. This data source was incredibly varied and complex. It needed to incorporate information like individual historical home and away face-off statistics; head-to-head matchup history; player characteristics such as height, weight, and handedness; and game context such as the location of the face-off, score of the game, and time of the face-off occurring.
This combination of historical success rates, player matchup characteristics, and game context brings together HITS and PPT data to provide a complete and unique perspective of face-off dynamics.
Adjusting for new face-off matchups
Face-off Probability is also flexible, adapting predictions based on changes to the game situation. For example, if a player is waived out of the face-off due to a violation, the model updates the prediction to the new matchup based on the real-time PPT streaming sensor data coming from the rink and from sensors attached to the players.
Predictions occur in less than a second and are triggered when there’s a change in players involved in the face-off. A prediction can also show the change in a team’s probability of winning a face-off when the primary center person is waived out of the face-off and replaced with a player with a different probability of success. This highlights the importance of having a particular player in the face-off circle. In addition to providing the probability of the two players facing off, the tool also provides other likely face-off combination pairings that will aid broadcast teams in highlighting potential matchups, which will enrich the viewing experience for fans.