Most of us don’t pay too much attention to the power grid that keeps our homes cooled and our businesses humming—until the lights go out or the refrigerator goes silent. But across California and the West Coast, as well as Australia and other drought-stricken parts of the world, energy companies are examining their infrastructures for risks beyond spoiled food, including wildfires. As fires get larger and increasingly occur outside of what we think of as fire season, being able to spot and fix issues before equipment failure occurs has become even more vital.
That was both the challenge and the opportunity San Diego Gas & Electric (SDG&E) put in front of its maintenance and digital innovation teams about two years ago. The solution they built with a team from Accenture—one that continues to evolve—is an elegant combination of the long experience of SDG&E’s qualified electrical workers, a bird’s-eye view of the utility’s equipment captured by a fleet of drones, and powerful machine-learning models running in the Amazon Web Services (AWS) Cloud.
In 2019, the initial idea being considered by SDG&E was to use drones to fly over about 75,000 of the utility’s 240,000 power poles—those in areas at highest risk of wildfire—to take images and identify equipment potentially in need of repair. When Jennifer Kaminsky, an SDG&E project manager overseeing large-transmission, distribution, and substation projects, heard about the idea of flying drones, she was intrigued by the possibility.
"I wanted to make sure it would amount to more than a lovely photo album of utility poles,” Kaminsky said.
It had to go beyond a neat way to gather images. The drone program had to help avoid the risk of wildfire. And to do that, Kaminsky and her team would need a mechanism that didn’t just take images, but could identify high-priority problems that required immediate attention.
Kaminsky is an expert when it comes to guiding utility projects through California’s numerous regulatory agencies and public and private stakeholders. She doesn’t fly drones for fun (“I have people for that,” she joked), but she had a very clear idea about the kind of data she wanted the drones to collect, at what level of detail, and what could be done with it.
Kaminsky pulled in an expert ally to help on the technology side—SDG&E’s Digital Strategy & Innovation Leader, Gabe Mika. When Mika heard drones were being put in the air as a proof-of-concept, he had the same instinct as Kaminsky: How could they do more with the imagery?
“I knew they were collecting the images, so the question was, ‘Could we apply computer-vision models to help us be more efficient long-term in our maintenance and fire prevention efforts?’,” Mika said. “Could we use the drone shots to build machine-learning models, and make this kind of drone program not just real, but something that had real benefits for our customers?”
For Kaminsky, real customer benefits can be defined by a straightforward idea.
“You fix things before they break,” she said.
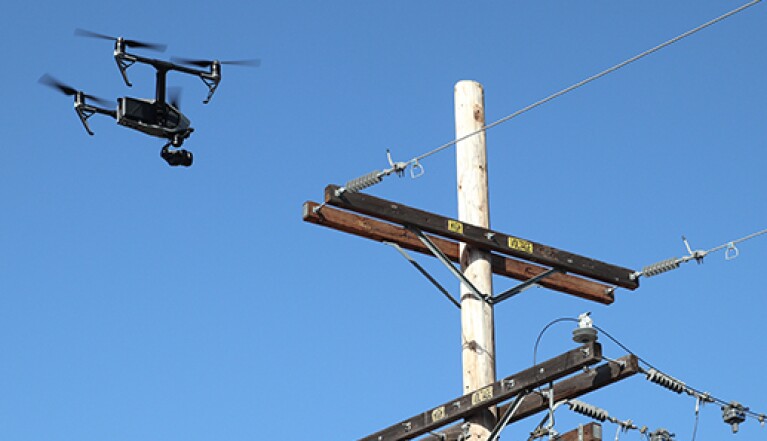
The utility business is often a very manual one. On a set schedule, a highly skilled crew inspect miles and miles of transmission and distribution lines—and, in the case of SDG&E, tens of thousands of poles—by literally stopping their trucks, looking up, taking notes, and logging them into a system.
Maintenance crews prioritize repairing the most critical and vulnerable parts of the system first, and then proceed down their list of less-urgent fixes. And then they do it all over again, all the while tackling the emergency fixes that inevitably come with weather events and assorted other unpredictable scenarios.
With the help of qualified drone pilots, Mika’s team, and the machine-learning advisors from Accenture, Kaminsky and SDG&E put drones in the air and started taking photos of the areas that were both hard to get to and at greatest risk: high fire-threat districts. SDG&E serves 3.6 million people in San Diego County and southern Orange County, so picture all the hillsides and sierras east and north of downtown San Diego. It’s rough, dry, and remote terrain; perfect, in other words, for flying a drone.
Kaminsky’s point of wanting more than just pictures anticipates the next task at hand: Developing image-recognition algorithms—machine-learning models—that could not only identify the transformers, insulators, arresters, cross-arms, and power poles they were looking at, but also determine the condition of the equipment and give it a score. The score would advise what images needed further review, and identify equipment that potentially needs fixing.
This is where the Accenture team’s deep experience in computer vision, data science, and developing applications to make it all work came to bear. They set about building a smart system that pulled in one more critical component: expert human experience.
The last part of what the system had to accomplish—gauging the condition of a piece of equipment—requires an expert eye and experience. Fortunately, that is exactly what SDG&E has in its linemen (officially known as qualified electrical workers), and it has made all the difference. As SDG&E’s Intelligent Image Processing program team describes it: Computer vision represents a human-plus-machine partnership toward asset inspections of the future.
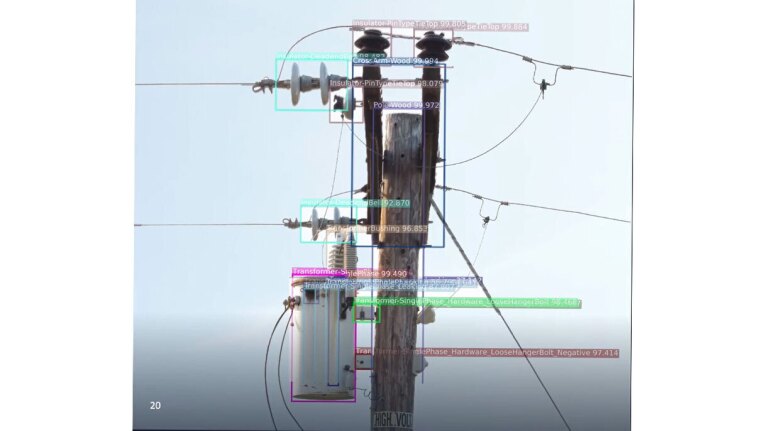
After teaching the image-recognition models to accurately identify the equipment by tagging, and confirming the contents of every single one of the 2.3 million photos—labeling the image data, in machine-learning parlance—SDG&E’s team applied their own experience to grading the condition of equipment. “Is that pole sound?” or “Is that lightning arrester blown or damaged?” aren’t always easy questions to answer, and the right answers come only by experience. By adding the insights of veteran SDG&E team members in the labeling phase, the dataset and the model become far more accurate when analyzing images. And if there is any ambiguity, the machine-learning model kicks it over to a human expert for further review and action. That might mean a follow-up physical inspection, or moving straight into a repair.
“Having the cloud has been a real game changer,” Kaminsky said. “Being able to store all of that data and have multiple users able to access it quickly, we have the potential to go from an assessment to a fix in the field very quickly.”
And she means it.
As part of the Innovation program that Mika leads, SDG&E uses a framework they call “learn, prove, scale.” The whole idea is to identify and pilot new solutions that make a material difference in speed and cost when solving for the utility’s toughest problems. Applying their framework, they kicked off the “learn” phase with drones and machine-learning-assisted image recognition. What they quickly found, which moved them into the “prove” stage, is that the speed that Kaminsky was looking for was real, and translated to real savings when calculating the commensurate time it would take for people to get in the field and make similar inspections.
Using Amazon Rekognition and Amazon Lookout for Vision, SDG&E’s machine-learning models can assess damage in close to 16,000 images an hour. In the near term, SDG&E plans to equip three dedicated vehicles and drivers with mobile devices and AWS machine-learning models running on the edge of the cloud to capture imagery in the high fire-threat district within 90 days. It would take the utility substantially longer to capture images of the same poles using drones. The idea is to scale this solution to more vehicles in their 1,500-vehicle fleet, to capture images across a majority of the service territory more frequently.
Two years and 2.3 million photos of transformers, insulators, connectors, and power poles later, the SDG&E team and their partner Accenture are now pushing hard to realize the transformational potential of the approach across the entire organization. That starts the thinking about the “scale” phase. How can they apply it not just to wildfire-risk reduction, but to asset management, vegetation management, and general reliability in a changing climate?
“The more varied the data we can get, the better we can train our models to do a variety of things,” Mika said. “Hopefully it gets to a point where we can share these out to the industry, because we share the same issues, and much of the same kinds of infrastructure. Wildfire is no longer a California thing—it's a West Coast thing, and it’s a global thing.”
The scale and intensity of the transformation that climate change is driving in communities and landscapes across the world require new ways of thinking and new methods to tackle what are novel problems. SDG&E’s efforts are a step towards that.
“It isn’t a fire season that begins and ends; it’s preparing for and preventing it by tracking weather patterns, measuring how much rain we get, and monitoring the moisture content of the vegetation,” Kaminsky said. “Fire science and climate adaptation are on our minds all year, and the more we look into this kind of data gathering and analysis, the more we believe it could be transformational not just for the utility industry, but also applicable to all kinds of other industries."
“Safety is our top priority, and by using human expertise combined with machine learning and these models, we can start to shine a light on things we didn’t see before,” she continued. “Things that help us prevent fires and keep the lights on for our customers.”